Data Mining: Why the Gold Rush?
My earlier post introduced the concept of data mining and highlighted some of the domains and applications that have utilized the set of technologies and expertise for their analytical needs. Many companies are accelerating towards including this toolset as a part of their decision making process. A casual survey of the current landscape seems to indicate that data mining has paid large dividends for many commercial and research enterprises.
At this point, you may be wondering about the reasons for this spike in interest in data mining techniques. The simple answer to the question is that there has been an exponential increase in the amount of data generated while the computational cost of storing and analyzing the data has decreased dramatically.
By some estimates, 2.5 billion gigabytes of data are generated each day globally, which represents an annual increase of 23% when measured over the last 2 decades. From Facebook “likes” to daily credit card transactions and cholesterol measurements, the span of data that is available for analysis is mind-boggling.
The argument therefore is that, with the availability of such data, reliance on human analysts and traditional techniques are no longer adequate to analyze the staggering breadth and complexity of data stores. Often, information is concealed in the data and is not readily evident by legacy analytical means or human intervention. Tools such as machine learning algorithms are better suited to search for complicated multi-factor patterns in the data without loss of objectivity. The cost of such automated algorithms is also much less expensive compared to employing additional analysts and statisticians.
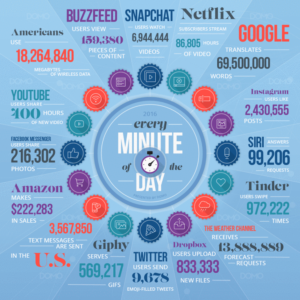
Finally, with the availability of such data, the competitive pressure faced by commercial organizations to transform the data into operational strategies and ultimately market share is immense. Retail companies are using data mining and machine learning algorithms to forecast product demand and tailor incentives and promotions at a customer level. Financial institutions are using similar tools to build individual credit risk profiles prior to authorizing lines of credit. While it is a little early to pronounce that the current state of data mining and machine learning is the panacea for all organizational issues, a recent survey by MIT Technology Review Custom and Google Cloud found that more than half of both early-stage and mature-stage users reported that deploying machine learning and other data mining techniques have resulted in demonstrable ROI.
Now that we know why organizations are jumping on the data mining bandwagon, it is time to delve a little deeper into some of the intricacies of these analytical tools. The next set of posts in this blog will try to address some of the techniques and their applications. Stay tuned!