Looking Beyond the Claim
Fraud is serious business. US healthcare payments and transactions eclipsed $1 trillion a year, and an estimated 10% of those payments are fraudulent. The Department of Justice estimates healthcare fraud costs US citizens a mind blowing $100 billion a year. The amount of zeros in these numbers is disheartening.
Finding fraud and eliminating it is a daunting task. Most fraud is detected once accumulated data reveals theft is taking place. This is changing thanks to a new era of machine learning powered software that can decrease the amount of data needed for fraud detection. However, fraud detection goes beyond data. Fraudsters game the system by manipulating the data they generate. On the surface, healthcare transactions show services and aid delivered to patients, but fraud is often only uncovered after investigating the details.
You see, fraudsters exhibit certain behaviors while gaming the healthcare system. The best anti-fraud agents, like ours here at Qlarant, combine behavioral science with data when combating fraud.
The Bigger Picture
Healthcare payments are transactional. A provider files a claim and that claim is paid by an insuring party. The insurer could be a major healthcare system, Medicaid, or Medicare. The transaction shows the dollars at risk for a claim. Smart data detectives look beyond transactions and search for key behavioral indicators (KBIs) fraudsters commit. Many fraudsters are sloppy with their paperwork and record keeping. Patients with incorrect personally identifiable information (PII), sharing PII across patients, and incorrect billing and profiles are common patterns. These kinds of fraudsters claim plausible deniability and incompetence through incorrect billing when they’re actually committing fraud. Other fraudsters game their patients through intimidation, others run scams on incorrect billing and refunds in a bid to antagonize patients.
Measuring KBIs, Feedback Loops
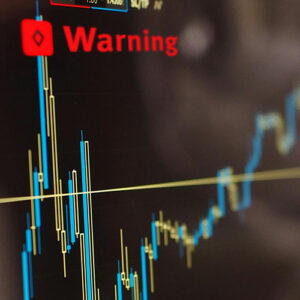
The challenge with adding KBIs to your anti-fraud toolkit is much of this information comes from other sources and must be aggregated with medical transactions. Collaborating with subject matter experts can help refine anti-fraud algorithms to minimize false positives and focus efforts towards the most egregious offenders.
Anti-fraud and risk intelligence software packages have to be adaptable and configurable so that KBIs can be a factor in the fraud detection feedback loop. Systems like RIViR are heavily adaptable, so when SMEs identify disruptive patterns in behavior and craft new KBIs, algorithms are updated delivering more accurate results.
In spite of their efforts to evade detection, fraud schemes and the bad actors behind them are constantly being identified and ultimately brought to justice through the efforts of anti-fraud agents and the tools they use. Those of us on the front lines, fighting fraud, are sharing knowledge, new techniques, and tools to make sure more people are getting the services they deserve and protecting benefits from scammers.